Genotype and Machine Learning Based Blood Matching: Towards Improved Transfusion Outcomes
10:30 til 12:30
Free admission
Date: Wednesday 2nd of April at 10:30 - 12:30 Hringsalur, Landspítali
The lecture begins at 11:00 but before light refreshments will be served.
Title: Genotype and Machine Learning Based Blood Matching: Towards Improved Transfusion Outcomes
Speaker: Nicholas Gleadall on behalf of the Blood Transfusion Genomics and Haem-Match
Consortium:
Assistant Professor of Transfusion and Transplantation Genomics
Victor Dahdaleh Heart and Lung Research Institute
Department of Haematology
University of Cambridge and NHS Blood and Transplant
Background
Blood transfusions save millions of lives worldwide each year, yet the formation of antibodies against non-self-antigens remains a significant problem, particularly in frequently transfused patients. The Blood Transfusion Genomics Consortium (BGC), a consortium of 15 major blood supply organisations, has developed a high-throughput genotyping technology for typing all clinically relevant blood cell antigens.
Concurrently, the Haem-Match Consortium has created machine-learning-based solutions to optimise blood allocation based on extended matching criteria. Together, these innovations provide blood services with the opportunity to improve transfusion outcomes for patients by reducing the risk of alloimmunisation while simultaneously optimising the logistics of blood supply.
Methods
The Blood Transfusion Genomics Consortium designed and tested the Universal Blood Donor Typing (UBDT) array for automated high-throughput simultaneous typing of human erythroid, platelet, and leukocyte antigens (HEA, HPA, and HLA). The study included 6,946 donors of European, African, Admixed American, South Asian, and East Asian ancestry, with genotyping being repeated in three different laboratories.
Concurrently, the Haem-Match Consortium developed the Blood Matcher framework to evaluate three matching strategies: (1) fully optimised antigen matching across all blood groups, (2) current guideline-based matching with exact ABO and D matching, and (3) a hybrid approach with exact ABO/D matching plus compatible matching for remaining antigens. Performance was assessed using 5,708 real-world blood requests from 911 patients across 11 NHSBT sites.
Results
The UBDT array demonstrated genotype reproducibility of ≥99% for 17,244 variants, translating to 99.98%, 99.90%, and 99.93% concordance across 338,372 HEA, 53,270 HPA, and 107,094 HLA genotypes, respectively. Compared to previous clinical typing data, concordance was 99.9% and 99.6% for 245,874 HEA and 3,726 HPA comparisons, while HLA types were 99.1% concordant with clinical typing. Beyond blood typing, hereditary hemochromatosis-associated HFE variants were identified in 276 donors. When applied to real-world allocation data (5,708 requests), the fully optimised matching algorithm fulfilled 99.95% of orders versus 84.39% with current guidelines while reducing overspecification of allocated units (18.9±2.7 vs. 20.4±4.5).
Simulation models predict that implementing these optimised algorithms with comprehensive genotyping data could reduce patient sensitisation risk by up to 80%, significantly decreasing adverse transfusion reactions among regularly transfused populations.
Conclusion
With NHSBT implementing donor genotyping and NHS England funding hemoglobinopathy patient genotyping in 2024, these complementary technologies can significantly improve transfusion outcomes, potentially reducing patient sensitisation risk by up to 80%. The UBDT array reliably types a wide range of blood cell antigens across diverse ancestries, significantly advancing improved patient care through extended matching. However, current guidelines limiting optimisation should be reevaluated to maximise patient benefits.
Nicholas Gleadall, Assistant Professor of Transfusion and Transplantation Genomics
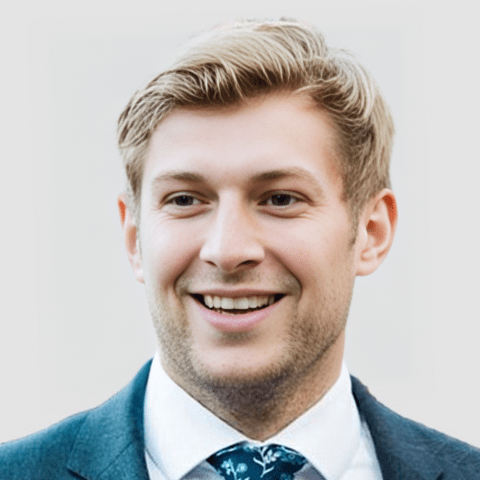